AI Model Fine-Tuning Myths: What You Need to Know
Understanding AI Model Fine-Tuning
AI model fine-tuning is a crucial step in customizing pre-trained models to suit specific tasks. While it offers remarkable potential, there are numerous myths surrounding the process that can lead to misconceptions. It’s important to understand these myths to make informed decisions when working with AI models.
Fine-tuning involves adapting a model that has already been trained on a large dataset to a new, smaller dataset. This process is key in enhancing the model’s performance for particular applications without the need to start training from scratch.
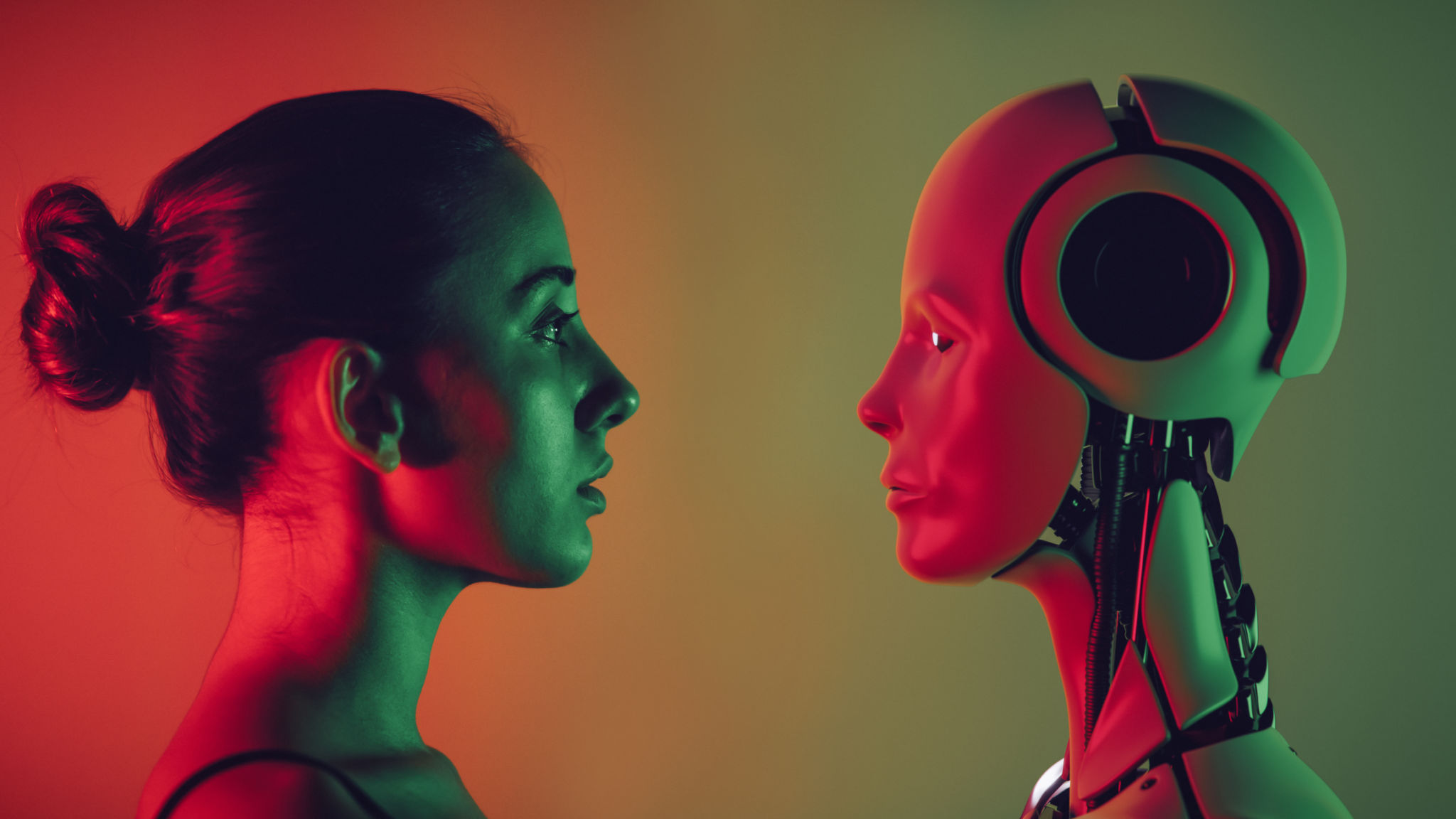
Myth 1: Fine-Tuning is Just for Big Companies
One common myth is that only large corporations with extensive resources can afford to fine-tune AI models. In reality, the democratization of AI tools has made fine-tuning accessible to smaller businesses and even individual developers. Open-source frameworks and cloud-based platforms offer affordable solutions for those looking to fine-tune models without the need for vast computational power.
Many platforms provide pre-trained models that can be easily adapted, making it a feasible option for a wide range of users. This accessibility is empowering companies of all sizes to leverage AI technologies effectively.
Myth 2: Fine-Tuning Requires Deep AI Expertise
Another misconception is that one must be an AI expert to successfully fine-tune a model. While having a foundational understanding of AI principles is beneficial, many tools and platforms are designed with user-friendliness in mind. They offer intuitive interfaces and step-by-step guidance, allowing individuals with varying levels of expertise to engage in the fine-tuning process.
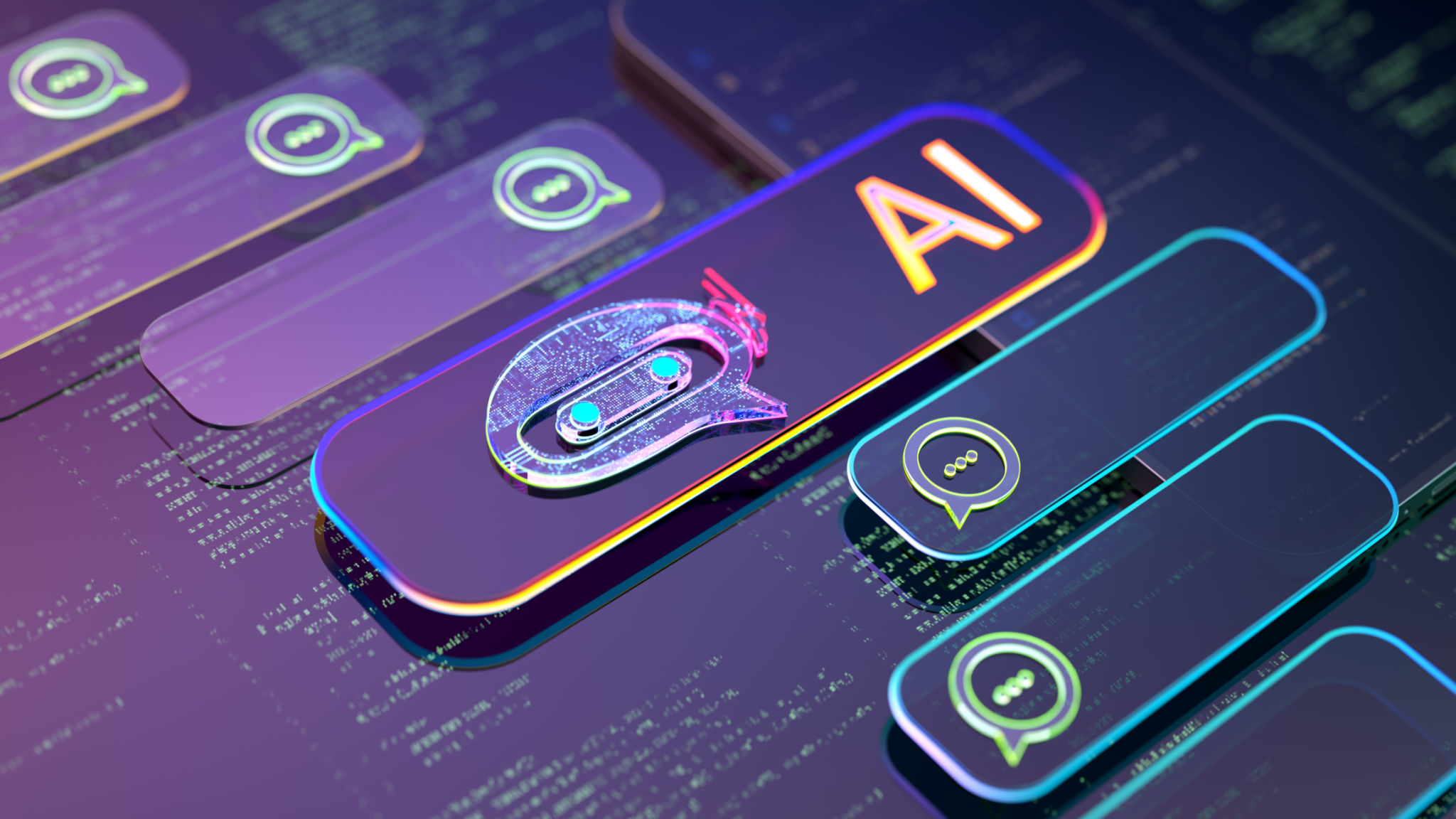
Moreover, numerous online resources and communities provide support and tutorials, making it easier than ever for non-experts to fine-tune models effectively.
Myth 3: Fine-Tuning Always Guarantees Better Results
It’s crucial to recognize that fine-tuning does not automatically guarantee improved results. The success of the process heavily depends on factors such as the quality of the new dataset, the relevance of the pre-trained model, and the specific requirements of the task at hand.
Before embarking on fine-tuning, it's essential to conduct thorough evaluations and experiments to validate whether fine-tuning will indeed enhance the model's performance for the desired application.
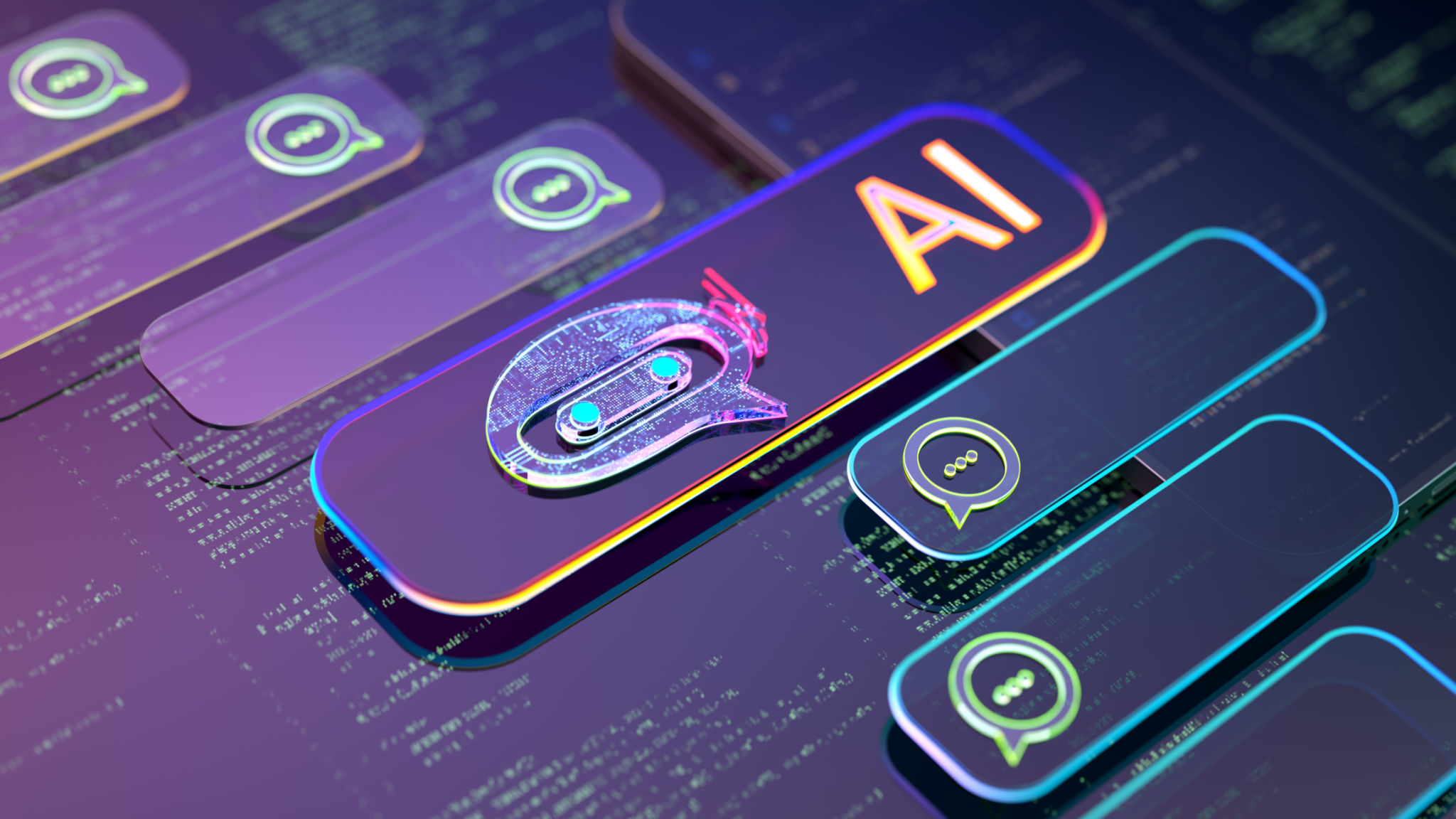
The Benefits of Dispelled Myths
By debunking these myths, businesses and developers can more confidently approach AI model fine-tuning. Understanding the realities allows for better planning, resource allocation, and outcome expectations. It opens up opportunities for innovation and efficiency that might have been overlooked due to misconceptions.
Fine-tuning is not just a tool for the tech giants but a versatile strategy available to everyone who seeks to optimize AI models for specific needs. Embracing this fact can lead to significant advancements in how AI is applied across various industries.
Conclusion
AI model fine-tuning holds immense potential for enhancing machine learning applications. By understanding and dispelling common myths, users can more effectively harness this powerful process. Whether you're a small business owner or an individual developer, fine-tuning offers opportunities to customize AI solutions that can drive innovation and efficiency in your ventures.