Experimenting with AI Model Fine-Tuning: Encouraging Innovation
Unlocking the Potential of AI Model Fine-Tuning
In the rapidly evolving field of artificial intelligence, fine-tuning models has become a critical strategy for enhancing performance and achieving specific outcomes. Fine-tuning allows developers to adjust pre-trained AI models so they align more closely with particular tasks or datasets. This process not only improves efficiency but also fosters innovation by enabling more customized applications.

The Basics of Fine-Tuning
Fine-tuning involves taking an existing model, often trained on a large dataset, and making incremental adjustments to its parameters based on a smaller, task-specific dataset. This process leverages the model's pre-existing knowledge while tailoring it to new challenges. The key advantage is that it requires less computational power and resources compared to training a model from scratch.
By employing techniques such as transfer learning, developers can effectively re-purpose models, allowing them to perform tasks like sentiment analysis, language translation, or even medical diagnosis with enhanced precision. This flexibility is crucial in industries that demand rapid adaptation to evolving requirements.
Encouraging Creative Applications
Fine-tuning AI models opens the door to a multitude of creative applications across various sectors. In healthcare, for instance, models can be adjusted to recognize patterns in medical images or predict patient outcomes with greater accuracy. Similarly, in finance, fine-tuned models can assist with risk assessment or fraud detection by analyzing transaction data more effectively.
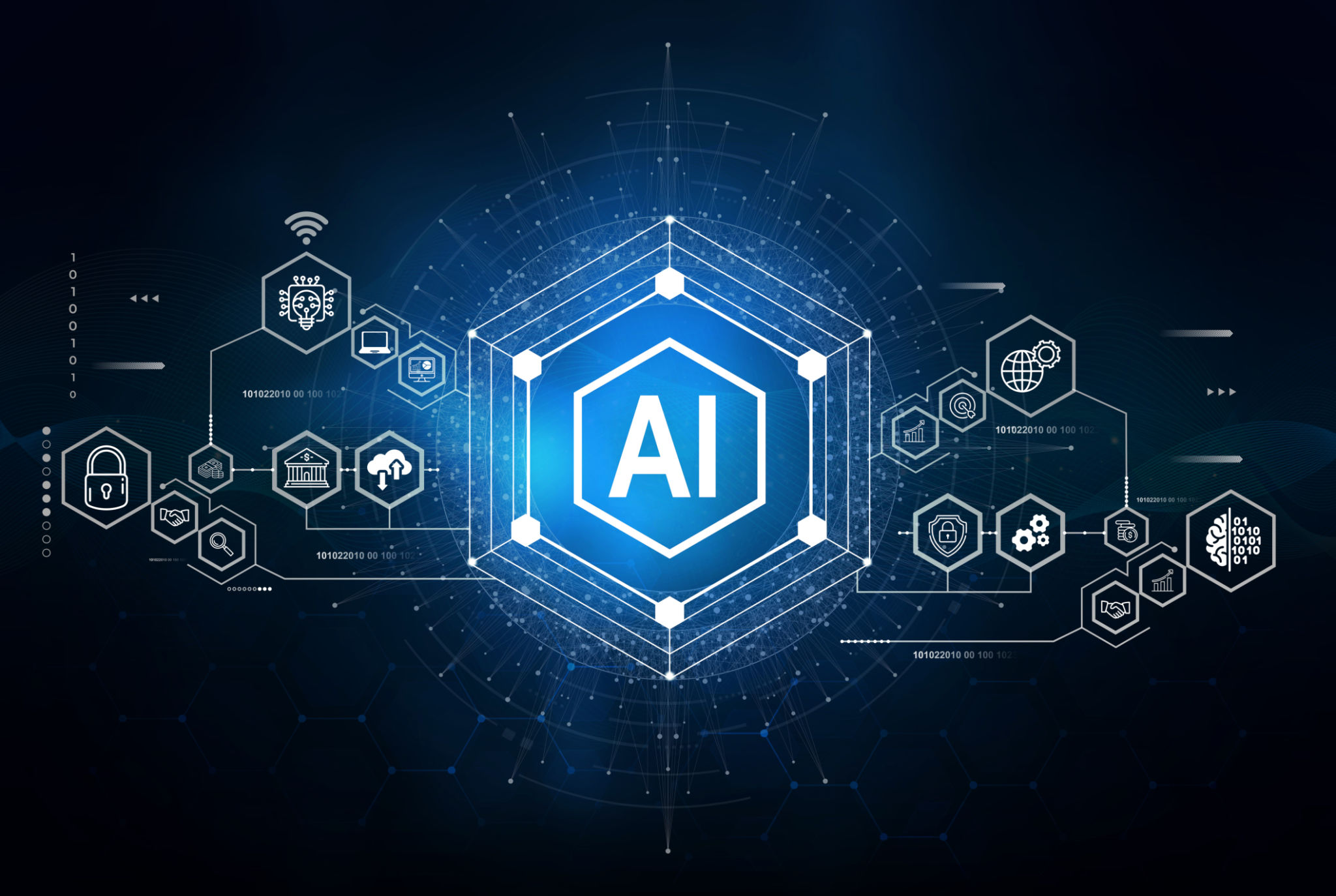
Moreover, in the realm of natural language processing (NLP), fine-tuned models can enhance chatbots and virtual assistants, making them more responsive and context-aware. This enables businesses to provide better customer service and improve user experiences significantly.
Challenges and Considerations
Despite its advantages, fine-tuning poses certain challenges that developers must consider. One significant issue is the risk of overfitting, where a model becomes too specialized for its fine-tuning dataset and loses its general applicability. To mitigate this, practitioners need to strike a balance between specificity and generalization.
Additionally, ethical considerations are paramount when fine-tuning AI models. Developers must ensure that models do not inadvertently learn biases present in the training data, which could lead to unfair or discriminatory outcomes. Rigorous testing and validation are essential steps in maintaining ethical standards.

The Future of Fine-Tuning
As AI technologies continue to advance, the potential for fine-tuning will expand even further. With ongoing research into methods like few-shot learning and meta-learning, the ability to customize models quickly and efficiently will become more accessible. This democratization of AI tools can empower smaller organizations and individual developers to innovate without needing massive resources.
The future of AI model fine-tuning promises not only improved technological capabilities but also a shift toward more personalized and adaptable solutions. By encouraging innovation through these practices, the possibilities for AI applications are virtually limitless.
In conclusion, fine-tuning is a powerful approach that holds immense potential for enhancing AI models' effectiveness across industries. By embracing this technique, developers can unlock new avenues for innovation and contribute to the growing landscape of intelligent solutions.